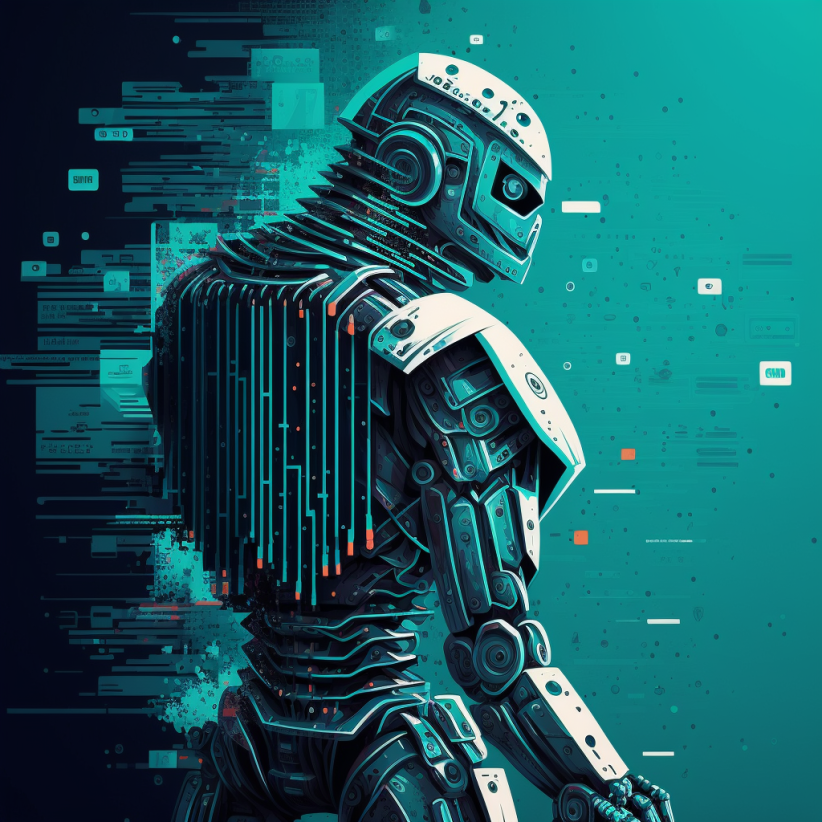
Understanding the most underrated trick in applied Machine Learning
Hashing is one of the most common “tricks” used in industrial Machine Learning applications, yet it doesn’t get nearly as much attention as it deserves.
The biggest advantage of hashing, especially in modern recommender systems, is its finite-memory guarantee: without hashing, it would be highly impractical to learn the relevance of billions of videos, news articles, photos, or web pages for billions of users without running out of memory.
But we’re getting ahead of ourselves here. This post is a primer, so let’s go back to where it all started: the famous 2009 “hashing trick” paper.
The paper that started it all
The idea of using hashing as a way to process features, as well as the term “hashing trick”, were first introduced in a 2009 paper by a team of researchers from Yahoo, led by Kilian Weinberger, in the context of Email spam detection. An email, after all, is a sequence of words, and…
…
Continue reading this article at;
https://towardsdatascience.com/hashing-in-modern-recommender-systems-a-primer-9c6b2cf4497a?source=rss—-7f60cf5620c9—4
https://towardsdatascience.com/hashing-in-modern-recommender-systems-a-primer-9c6b2cf4497a?gi=96e10d7ac258&source=rss—-7f60cf5620c9—4
towardsdatascience.com
Feed Name : Towards Data Science – Medium
editors-pick,hashing,recommender-systems,data-science,machine-learning
hashtags : #Hashing #Modern #Recommender #Systems #Primer #Samuel #Flender